
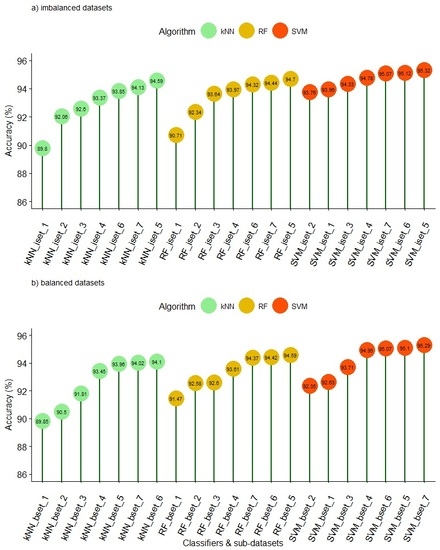
Rosen, “Selecting age-related functional characteristics in the human gut microbiome,” Microbiome, 2013, 1(1), 1-12. Russell, “Variation of symbiotic gut communities across diets and colonies of the ant Cephalotes varians,” in Entomological Society of America Annual Meeting, 2012. The analysis of these diverse datasets uncovered microbiome alternation over various courses, including the consequences of symbiotic evolution, during human aging or environmental changes. Next-generation sequencing data analysis applied on various topics: during the development of tools and databases, I gained access to a variety of next-generation sequencing data. Hershberg, “Marker genes that are less conserved in their sequences are useful for predicting genome-wide similarity levels between closely related prokaryotic strains,” Microbiome, 2016, 4(1), 1. Rosen, “POGO-DB-a database of pairwise-comparisons of genomes and conserved orthologous genes,” Nucleic Acids Research, 2014, 42(D1), D625-D632. It features a web interface and API access, allowing users to compare and select optimal marker genes that can better resolve the phylogenetic relationships between closely related microbes. Using high-performance computation to compare all existing prokaryotic whole genomes, I developed POGO-DB, a database of pairwise comparison of genomes and universal orthologous genes. While alternative marker genes were used occasionally, it was difficult to compare and decide the best marker gene in various scenarios. Rosen, “Fizzy: feature subset selection for metagenomics,” BMC Bioinformatics, 2015, 16(358).Ĭonstruction of genomic database: for decades the scientific community has been using single marker genes to identify microbial organisms and lived with the fact that the most popular marker gene (16S rRNA gene) could not well differential microbes at the species level. Rosen, “Feature selection for metagenomic data analysis,” Encyclopedia of Metagenomics, 2014, Ed: K. Rosen, “Advances in machine learning for processing and comparison of metagenomic data,” Computational Systems Biology: From Molecular Mechanisms to Disease, 2013, Ed: A. Rosen, “Using the RDP classifier to predict taxonomic novelty and reduce the search space for finding novel organisms,” PLOS ONE, 2012, 7(3), e32491.
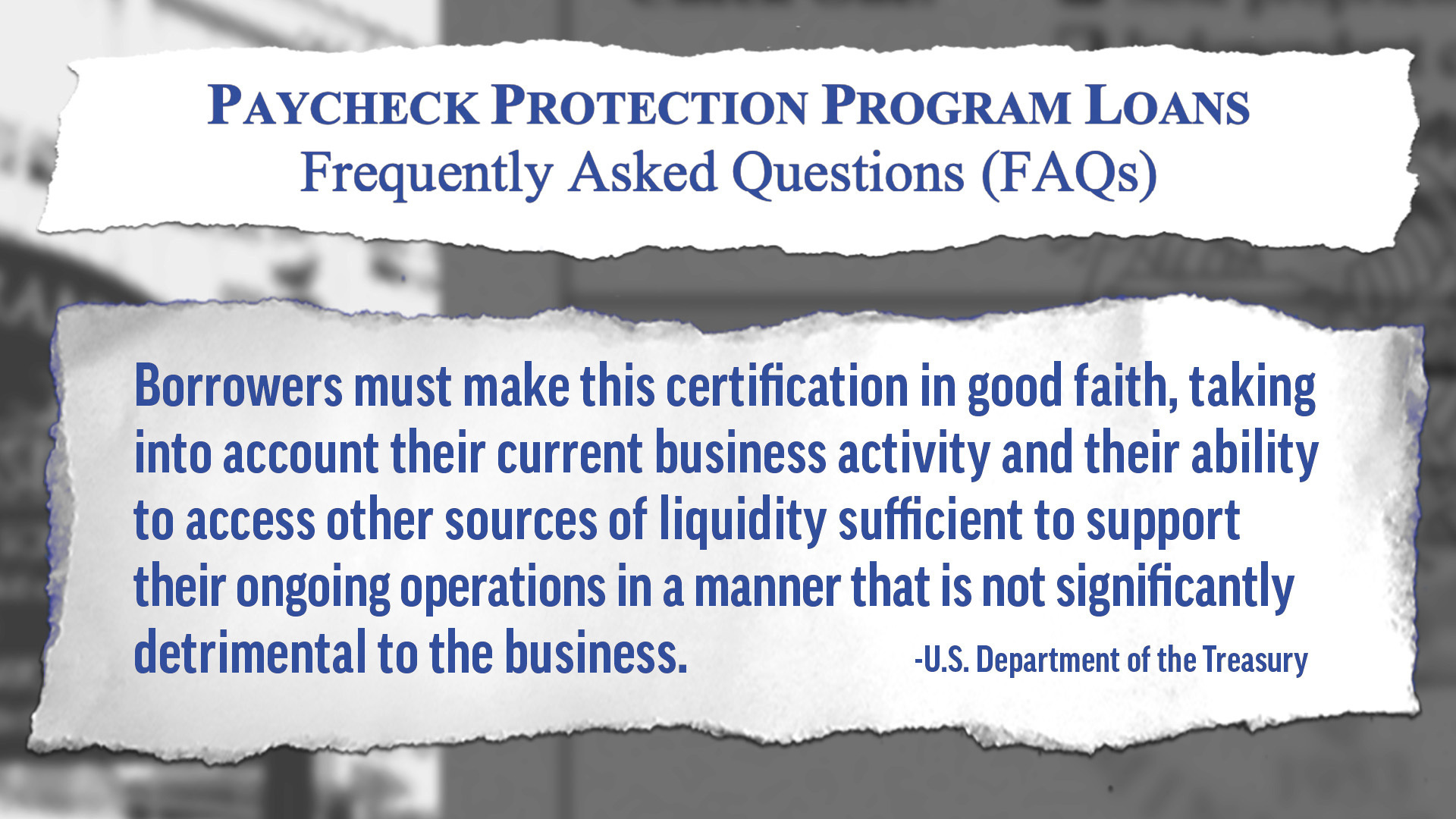
I also collaborated with the Ribosomal Database Project research group, and established a set of standards for their taxonomic classification tool. This algorithm has been packaged as the FIZZY software. gene sets) most meaningful in comparative genomics studies.
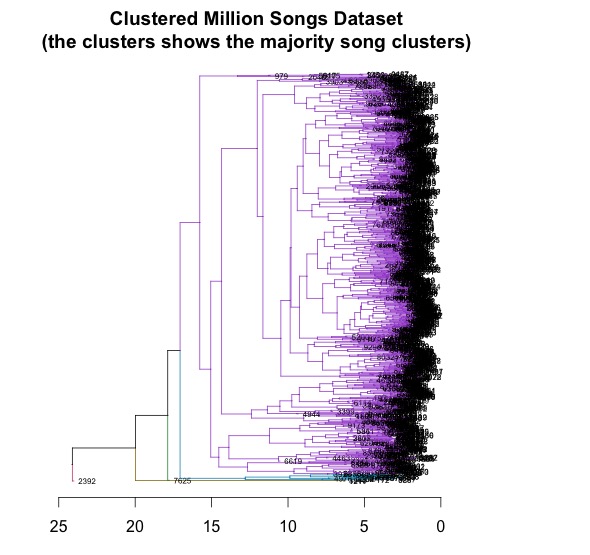
I integrated feature selection algorithms and supervised classification to identify signatures (e.g.
#Drexel university million song dataset how to#
One of the intriguing challenge’s in bioinformatics as well as other big data analysis was, how to extract meaningful information from a large amount of information. Rosen’s lab, my early research interest was to develop computational algorithms for bioinformatics analysis.
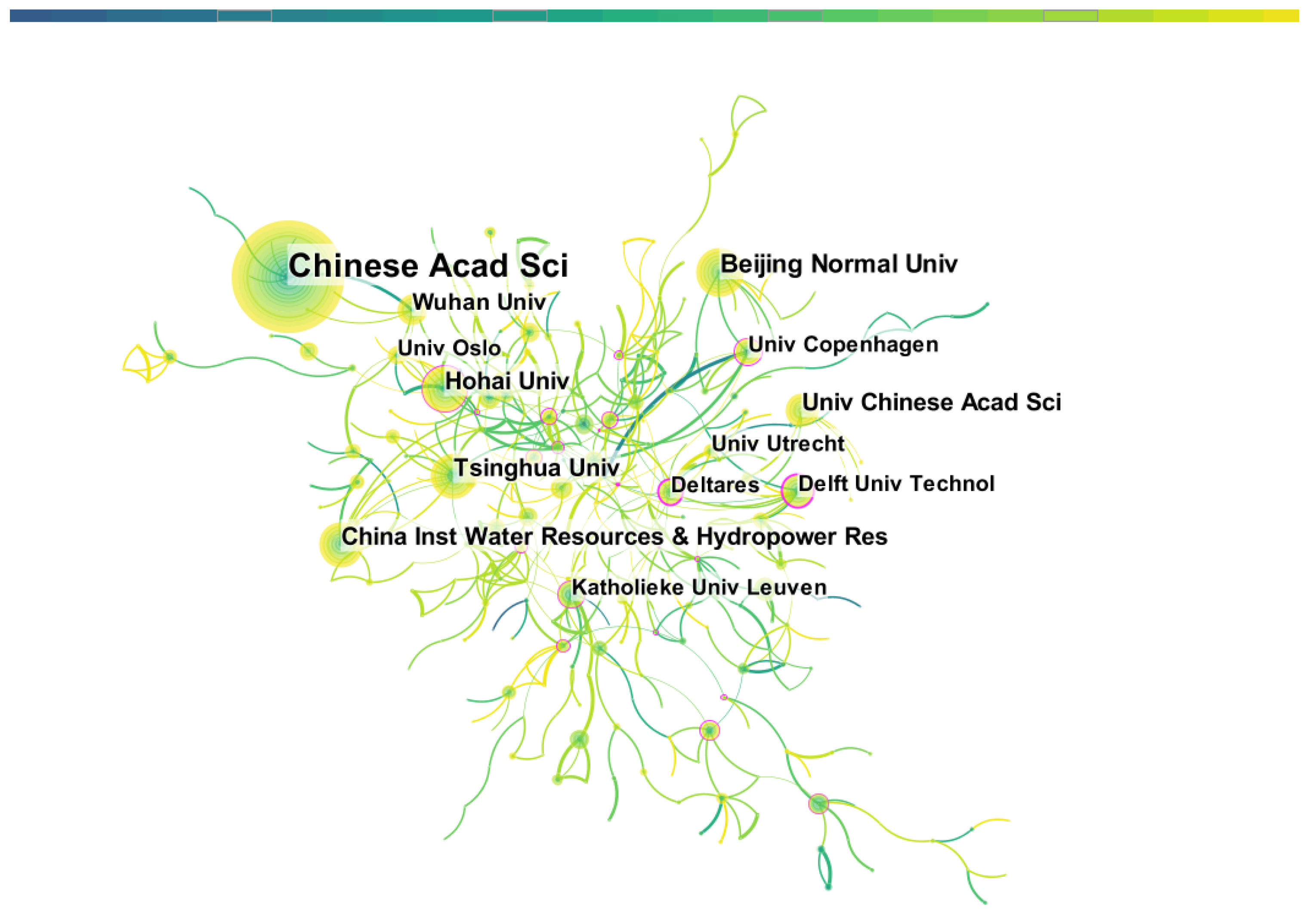
Development of bioinformatics tools: as a PhD candidate in Dr.
